Segmentation of prior austenite grains in steel
Task definition:
- Determining the prior austenite grain size of a steel has been a long-standing challenge. Established approaches (e.g. chemical etching with Bechet-Beaujard or crystallographic reconstruction using electron backscatter diffraction (EBSD)) have limitations specific to their processes
- A Deep Learning (DL) model is to be trained to robustly segment the prior austenite grains (PAG) in microstructures after Bechet-Beaujard etching, despite the occurance of etching artefacts
Additionally, another DL model is to be trained to segment the PAG in microstructures etched with regular nital under specific conditions
Realization:
- Use of DL (semantic segmentation)
- Training separate models for Bechet-Beaujard and Nital etching
- Use of correlative microscopy (LM/SEM and EBSD) for objective ground truth

Segmentation example for austenite grains
Results:
- Very good model performance for both Bechet-Beaujard and Nital etching
- Good segmentation even with new, unseen images


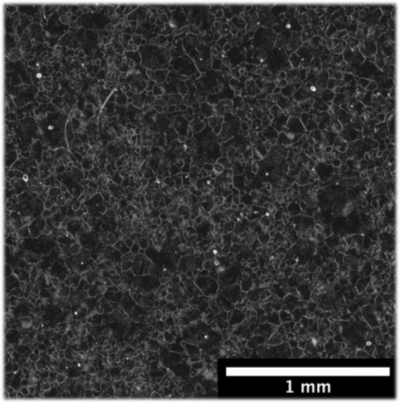
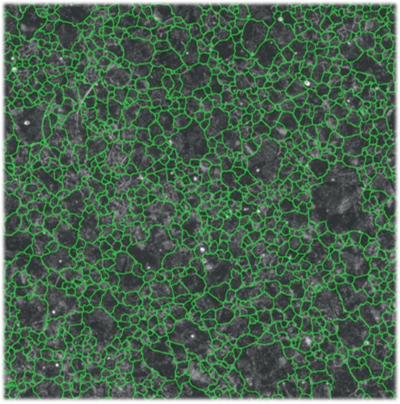
Segmentation of Bechet-Beaujard images


Segmentation of Nital images
Fields of application:
- Automated, objective and reproducible determination of the prior austenite grain size
Unlike the often-manual evaluation methods (e.g., comparative grain size according to the line cut method), this approach enables determination of the grain size distribution
- Increased efficiency: Quick quantification of even larger sample areas (e.g. 2 mm x 2 mm)
- Time saving potential by substituting EBSD reconstruction
- Improved microstructural analysis as a basis for process-structure property correlations
Partners of cooperation:
- Joint-stock company of Dillinger Huettenwerke, Dillingen/Saar
References:
- Bachmann, B. I., Müller, M., Britz, D., Durmaz, A. R., Ackermann, M., Shchyglo, O., Staudt, T., & Mücklich, F. (2022). Efficient reconstruction of prior austenite grains in steel from etched light optical micrographs using deep learning and annotations from correlative microscopy. Frontiers in Materials, 9(October), 1–18. https://doi.org/10.3389/fmats.2022.1033505
- Laub, M., Bachmann, B.-I., Detemple, E., Scherff, F., Staudt, T., Müller, M., Britz, D., Mücklich, F., & Motz, C. (2022). Determination of grain size distribution of prior austenite grains through a combination of a modified contrasting method and machine learning. Practical Metallography, 60(1), 4–36. https://doi.org/10.1515/pm-2022-1025
Contact

Dr.-Ing. Dominik Britz
Deputy head of MECS Saarbrücken
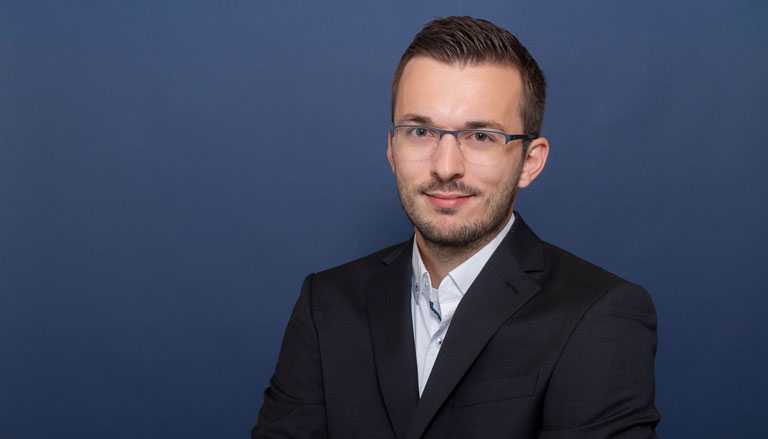
Adrian Thome, M.Sc.
Chief Operating Officer
You are interested in working with us?
Feel free to contact us! We look forward to talking to you and finding out how we can help you with your project.