Machine learning

DL Segmentation of lath-shaped bainite – LM*
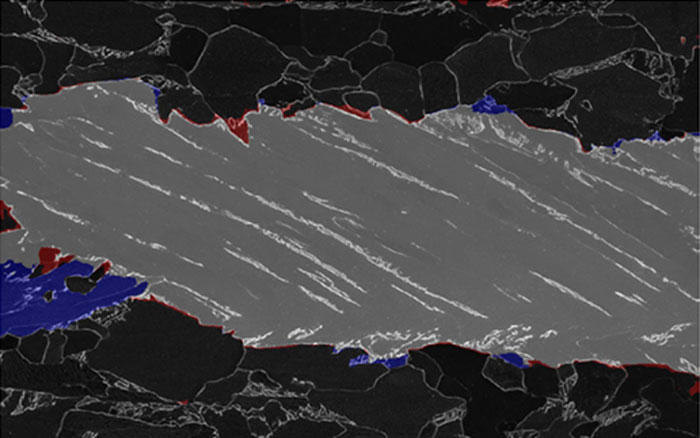
DL Segmentation of lath-shaped bainite – SEM*
Benefits of Machine Learning (ML)
- Presents promising new possibilities in microstructure analysis
- Automates of time-consuming and labour-intensive standard tasks
- Facilitates the evaluation of large, high-dimensional data volumes
- Ensures objectivity and reproducibility
- Serves as foundation for improved analyses of complex microstructures previously unattainable
How we approach ML
- Computer science expertise is blended with domain knowledge in material science
- A holistic approach to ml is adopted: including all the steps involved in obtaining a microstructure image.
- This differentiates from approaches by pure data scientists who may overlook domain knowledge
- Emphasis is placed on accurately assigning ground truth for supervised ML.
- Priority is given to controlling and understanding material science-related aspects, recognizing their significance for success over solely optimizing ML parameters
Contact

Dr.-Ing. Dominik Britz
Deputy Head MECS Saarbrücken
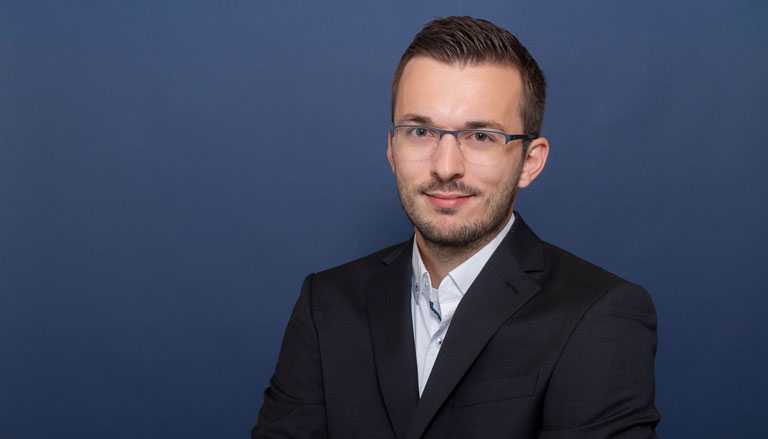
Adrian Thome, M.Sc.
Chief Operating Officer
Our services offered:
- Customised ML-based solutions for automated microstructure analysis
- Focus on objective ground truth, e.g. through correlative microscopy
- Holistic approach: metallographic boundary conditions required for successful ML implementation
- Customised training (ML basics, ML in materials science and engineering, ML in metallography and microstructural analysis)
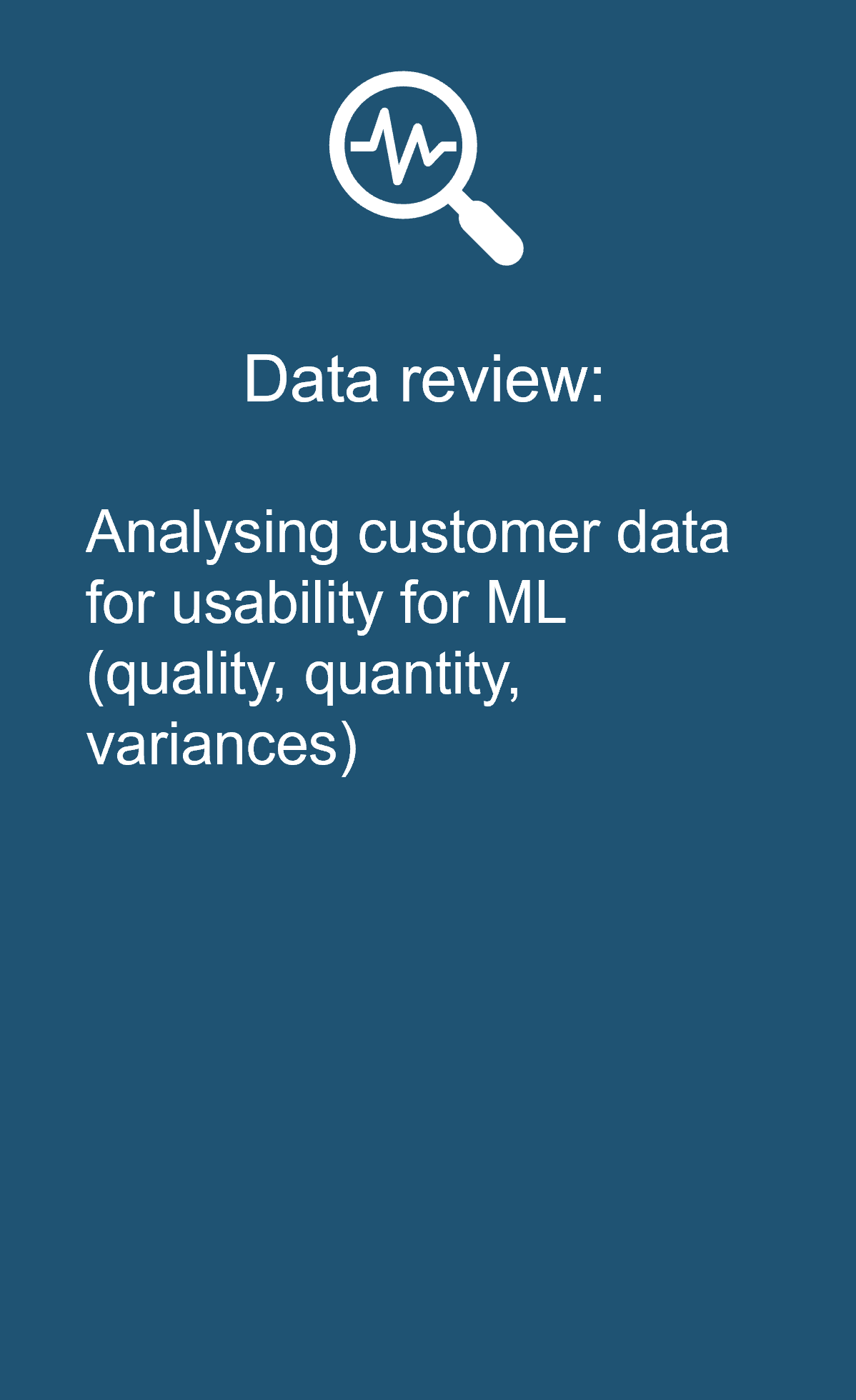
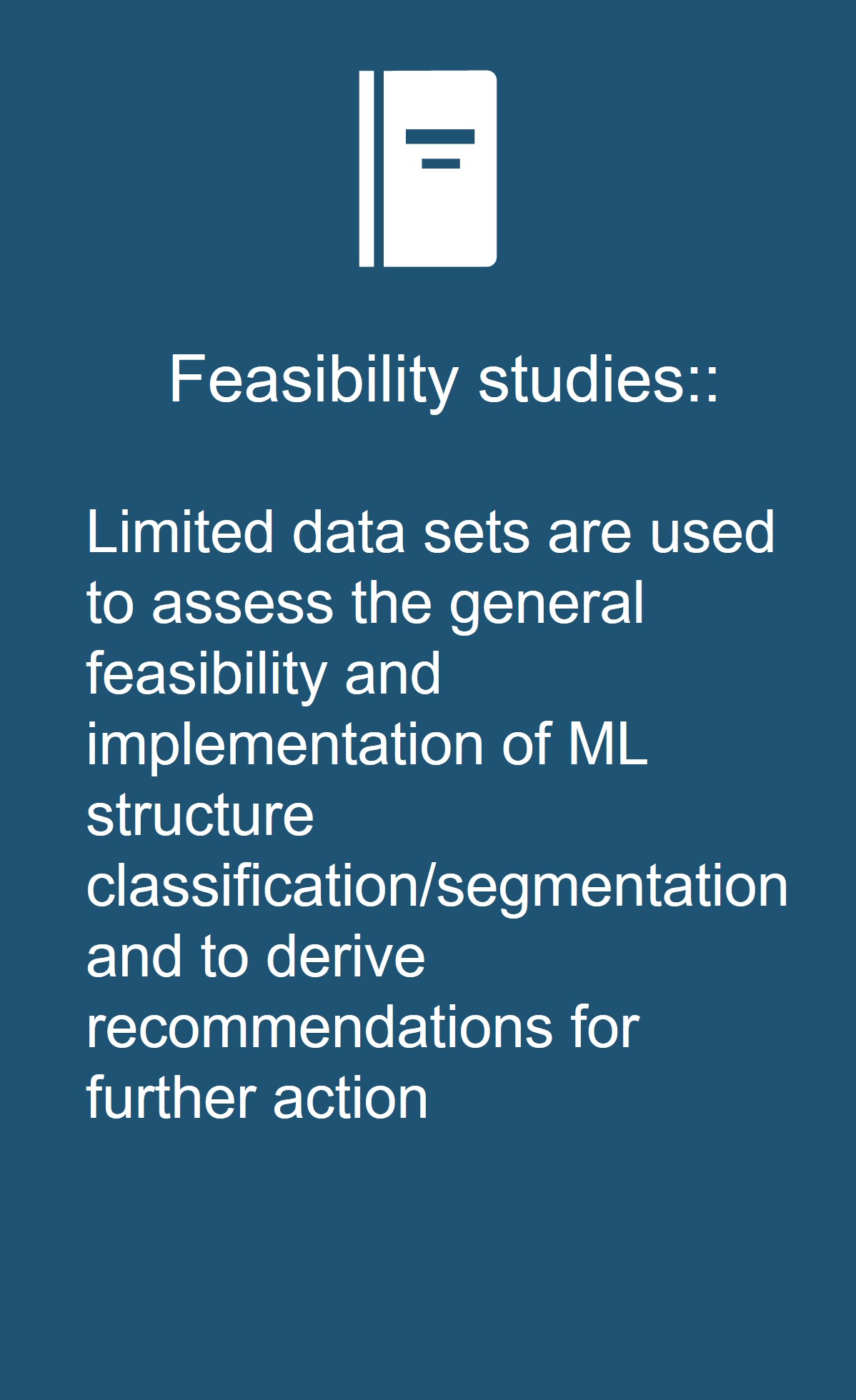
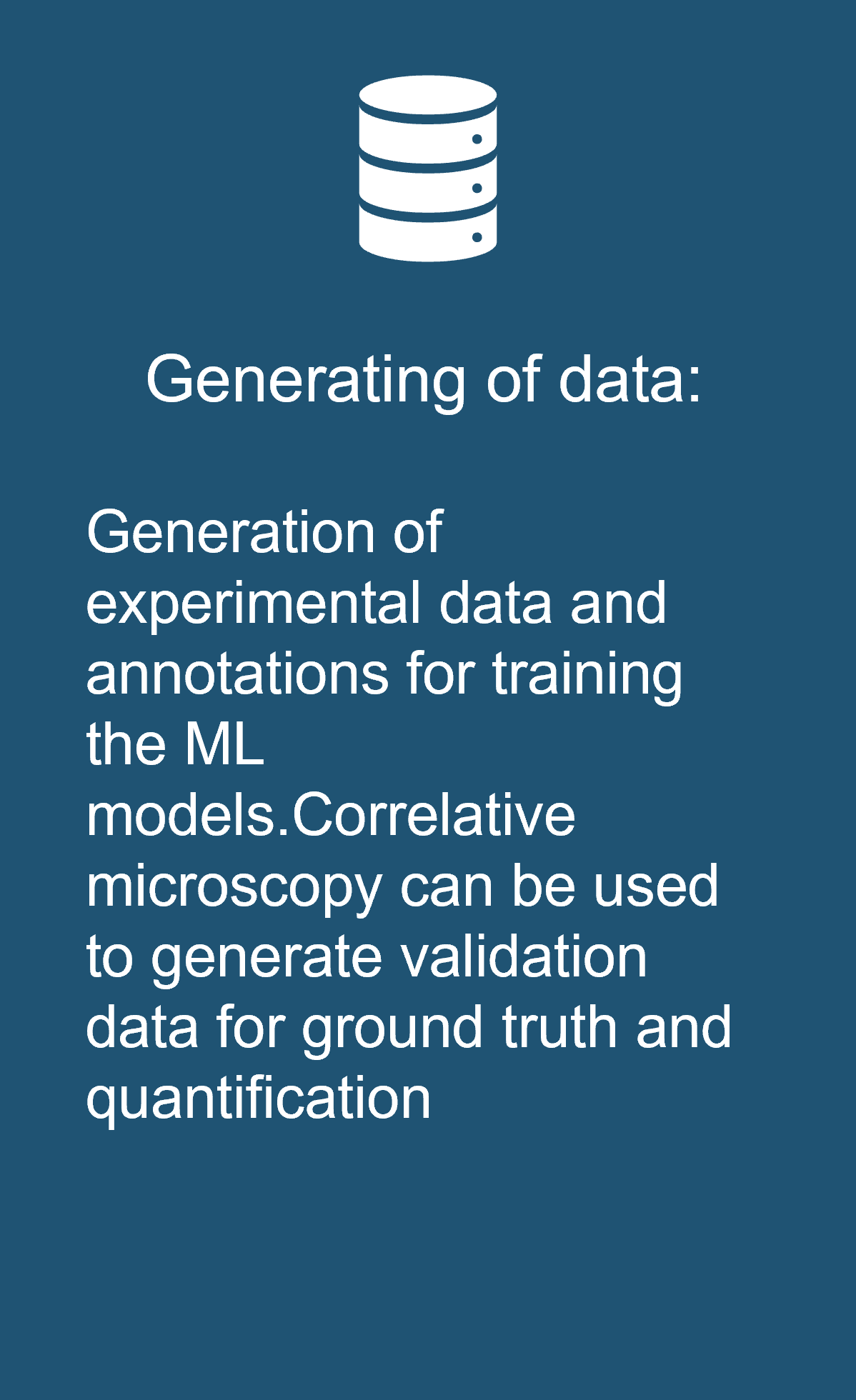
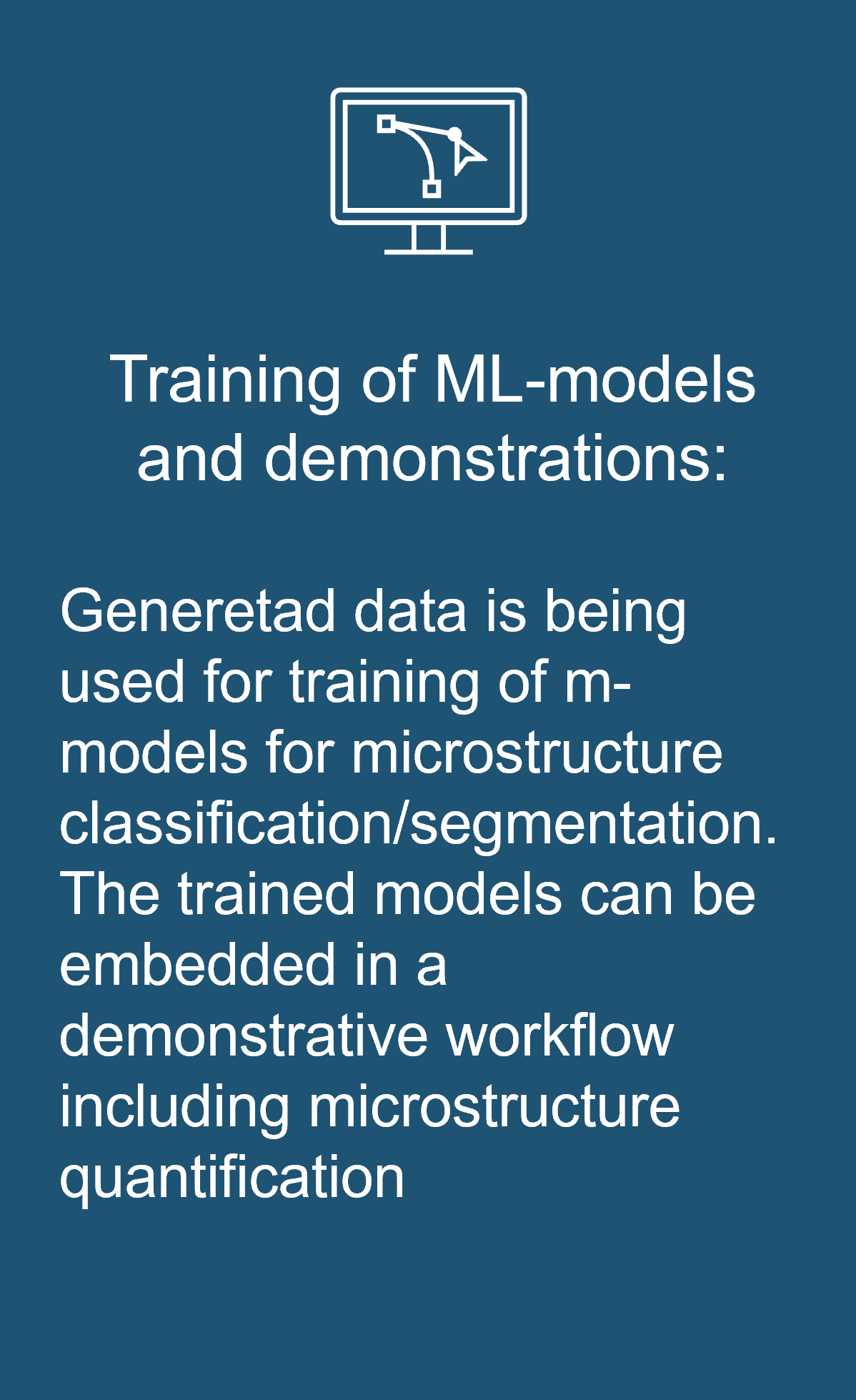
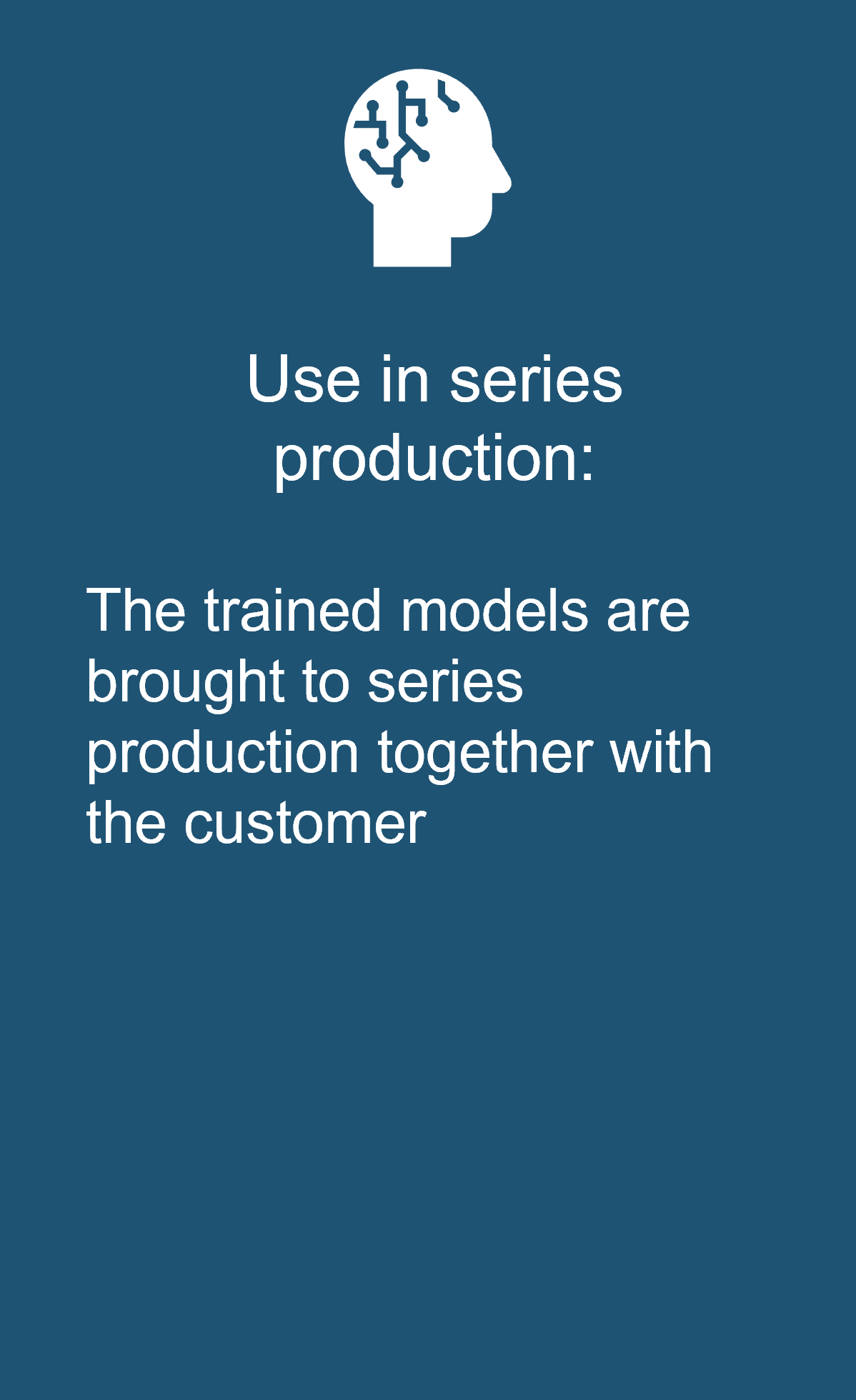
Where our ML approaches are successfully applied
In addition to standard everyday metallographic tasks, such as grain size determination, phase analysis, layer thickness measurement or fracture surface analysis, our ML approaches are used in particular for complex microstructural analyses for which no conventional solutions were previously available.
Segmentation of lath-shaped bainite in multiphase steels
Microstructure classification in heat-treatable steels
Classification of non-metallic inclusions
Classification of the carbon-rich second phase in dual-phase steels
Segmentation of former austenit grains in steel
Reference projects
Joint stock company of Dillinger Hüttenwerke
- Strategic partnership:
Establishment of ML methods in microstructure analysis - Key figures:
- 4 doctoral projects
> 30 student theses
> 20 national and international conference papers
> 20 publications
- 4 doctoral projects
Further areas of application
Correlative Microscopy
A correlative characterisation of microstructures serves as a benchmark for automated microstructure recognition and as a starting point for machine learning approaches.
Triboelectric characterisation
In addition to our microstructure analysis, we are able to carry out various triboelectric measurements (e.g. electrical resistance and friction coefficient in the mating test) under controlled conditions.
Failure Analysis
In the field of failure analysis, we are not only able to identify and analyse fracture soles but also to fully characterise components on a macro and micro scale.
Antimicrobial surfaces
Our antimicrobial metallic surfaces have already been used in 3 research projects with ESA & NASA on the ISS.
Welding technology consulting
We assess the suitability of materials for welding and make suggestions for the use of materials, welding consumables and welding processes